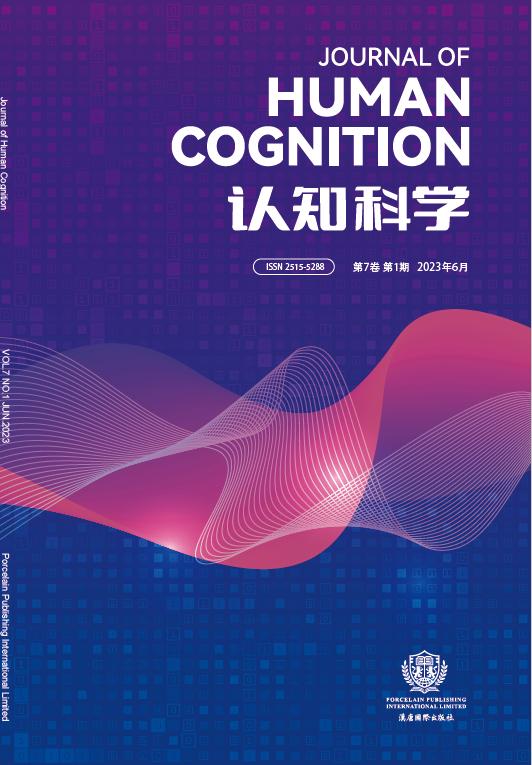
Volume 7, Issue 1
创造力、涌现能力与经验主义的胜利
- 创造力、涌现能力与经验主义的胜利
- Vol. 7, Issue 1, Pages: 29-43(2023)
Published: 30 June 2023
DOI:10.12184/wsprzkxWSP2515-528802.20230701
Scan QR Code
Volume 7, Issue 1
山西大学哲学学院,山西太原,030006
Published: 30 June 2023 ,
Scan QR Code
单位.创造力、涌现能力与经验主义的胜利[J].认知科学,2023,07(01):29-43.
徐超. (2023). 创造力、涌现能力与经验主义的胜利. Cognitive Scienc, 7(1), 29-43..
单位.创造力、涌现能力与经验主义的胜利[J].认知科学,2023,07(01):29-43. DOI: 10.12184/wsprzkxWSP2515-528802.20230701.
徐超. (2023). 创造力、涌现能力与经验主义的胜利. Cognitive Scienc, 7(1), 29-43.. DOI: 10.12184/wsprzkxWSP2515-528802.20230701.
ChatGPT的发布引发了哲学界的广泛热议,学界对于ChatGPT是否在技术层面有本质创新持有不同的意见。林田博士与任晓明教授的《对话机器、深度学习与人工智能“新经验主义革命”的功与过》一文通过系统地对比规则导向和数据导向两种主流技术路线的发展史,从哲学史的视角对“新经验主义革命”的功与过进行评价。靶文认为ChatGPT并未带来技术层面的本质创新,其回答纯粹来自人类语料里已有的内容,只是解决了经验论一贯善于解决的问题,同时认为这种解决方案是有代价的且存在缺陷的,远不是强人工智能或通用人工智能,因此,并不能代表经验主义的胜利。本文主要就以下三个问题与作者商榷:1)ChatGPT是否具有创造力,其回答是否纯粹来自人类语料里已有的内容;2)如何看待ChatGPT的涌现能力,其是否只能解决传统经验论一贯善于解决的问题;3)ChatGPT是否意味着经验主义的胜利。
ChatGPT创造力涌现能力经验主义
Borji A. (2023). A categorical archive of ChatGPT failures. arXiv preprint arXiv:2302.03494.
Brown T., Mann B., Ryder N., Subbiah M., Kaplan J. D., Dhariwal P., et al. (2020). Language models are few-shot learners. Advances in Neural Information Processing Systems, 33:1877–1901.
Bubeck S., Chandrasekaran V., Eldan R., Gehrke J., Horvitz E., Kamar E., et al. (2023). Sparks of artificial general intelligence: Early experiments with GPT-4. arXiv preprint arXiv:2303.12712.
Chen M., Tworek J., Jun H., Yuan Q., Pinto H. P. d. O., Kaplan J., et al. (2021). Evaluating large language models trained on code. arXiv preprint arXiv:2107.03374.
Chowdhery A., Narang S., Devlin J., Bosma M., Mishra G., Roberts A., et al. (2022). PaLM: Scaling language modeling with pathways. arXiv preprint arXiv:2204.02311.
Fu, Yao; Peng, Hao and Khot, Tushar. (2022). How does GPT Obtain its Ability? Tracing Emergent Abilities of Language Models to their Sources. Yao Fu's Notion. https://yaofu.notion.site/How-does-GPT-Obtain-its-Ability-Tracing-Emergent-Abilities-of-Language-Models-to-their-Sources-b9a57ac0fcf74f30a1ab9e3e36fa1dc1https://yaofu.notion.site/How-does-GPT-Obtain-its-Ability-Tracing-Emergent-Abilities-of-Language-Models-to-their-Sources-b9a57ac0fcf74f30a1ab9e3e36fa1dc1
Goodfellow I., Bengio Y., and Courville A. (2016). Deep Learning. MIT Press.
Jiang A. Q., Li W., Tworkowski S., Czechowski K., Odrzygóźdź T., Miłoś P., et al. (2022). Thor: Wielding hammers to integrate language models and automated theorem provers. Advances in Neural Information Processing Systems, 35:8360–8373.
Kıcıman E., Ness R., Sharma A., and Tan C. (2023). Causal reasoning and large language models: Opening a new frontier for causality. arXiv preprint arXiv:2305.00050.
Kojima T., Gu S. S., Reid M., Matsuo Y., and Iwasawa Y. (2023). Large language models are zero-shot reasoners. arXiv preprint arXiv:2205.11916.
LeCun Y., Bengio Y., and Hinton G. (2015). Deep learning. Nature, 521(7553):436–444.
Li H. (2017). Deep learning for natural language processing: Advantages and challenges. National Science Review, 5(1):24–26.
OpenAI. (2023). GPT-4 technical report.
Radford A., Wu J., Child R., Luan D., Amodei D., Sutskever I., et al. (2019). Language models are unsupervised multitask learners. OpenAI Blog.
Saba W. S. (2018). A simple machine learning method for commonsense reasoning? A short commentary on Trinh & Le (2018). arXiv preprint arXiv:1810.00521.
Sun Y., Wang S., Feng S., Ding S., Pang C., Shang J., et al. (2021). ERNIE 3.0: Large-scale knowledge enhanced pre-training for language understanding and generation. arXiv preprint arXiv:2107.02137.
Tu S., Li C., Yu J., Wang X., Hou L., and Li J. (2023). ChatLog: Recording and analyzing chatgpt across time. arXiv preprint arXiv:2304.14106.
Wang S., Sun Y., Xiang Y., Wu Z., Ding S., Gong W., et al. (2021). ERNIE 3.0 Titan: Exploring larger-scale knowledge enhanced pre-training for language understanding and generation. arXiv preprint arXiv:2112.12731.
Wei J., Tay Y., Bommasani R., Raffel C., Zoph B., Borgeaud S., et al. (2022). Emergent abilities of large language models. arXiv preprint arXiv:2206.07682.
Wei J., Wang X., Schuurmans D., Bosma M., Chi E., Le Q., and Zhou D. (2023). Chain of thought prompting elicits reasoning in large language models. arXiv preprint arXiv:2201.11903.
Wu Y., Jiang A. Q., Li W., Rabe M., Staats C., Jamnik M., and Szegedy C. (2022). Autoformalization with large language models. Advances in Neural Information Processing Systems, 35:32353–32368.
Zhang C., Zhang C., Li C., Qiao Y., Zheng S., Dam S. K., et al. (2023). One small step for generative AI, one giant leap for AGI: A complete survey on ChatGPT in AIGC era. arXiv preprint arXiv:2304.06488.
Zheng K., Han J. M., and Polu S. (2022). miniF2F: A cross-system benchmark for formal olympiad-level mathematics. In Proceedings of the International Conference on Learning Representations.
林田,任晓明. (2023). 对话机器、深度学习与人工智能"新经验主义革命"的功与过. 认知科学.
徐超. (2023). 基于认知的自然语言自动形式化研究. 中国社会科学出版社.
Related Articles
Related Author
Related Institution