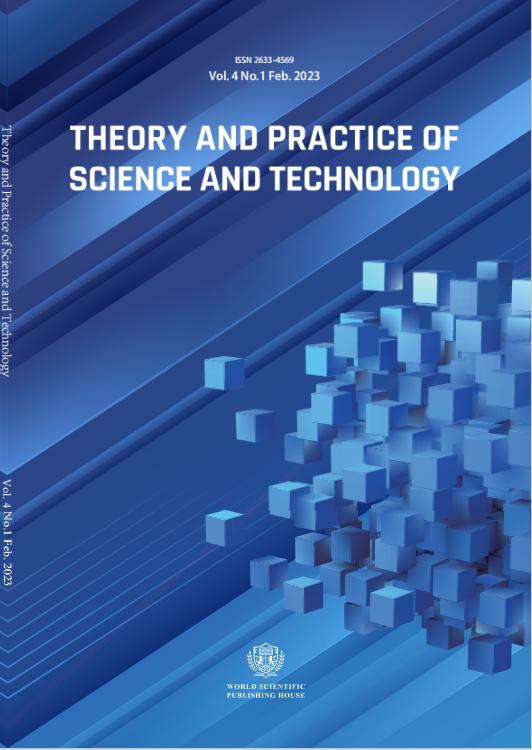
4卷 第1期
The Solution of Cold Start Problem in Recommender System Introduction
The Solution of Cold Start Problem in Recommender System Introduction
- 2023年4卷第1期 页码:48-54
纸质出版日期: 2023
DOI:10.47297/taposatWSP2633-456908.20230401
Scan QR Code